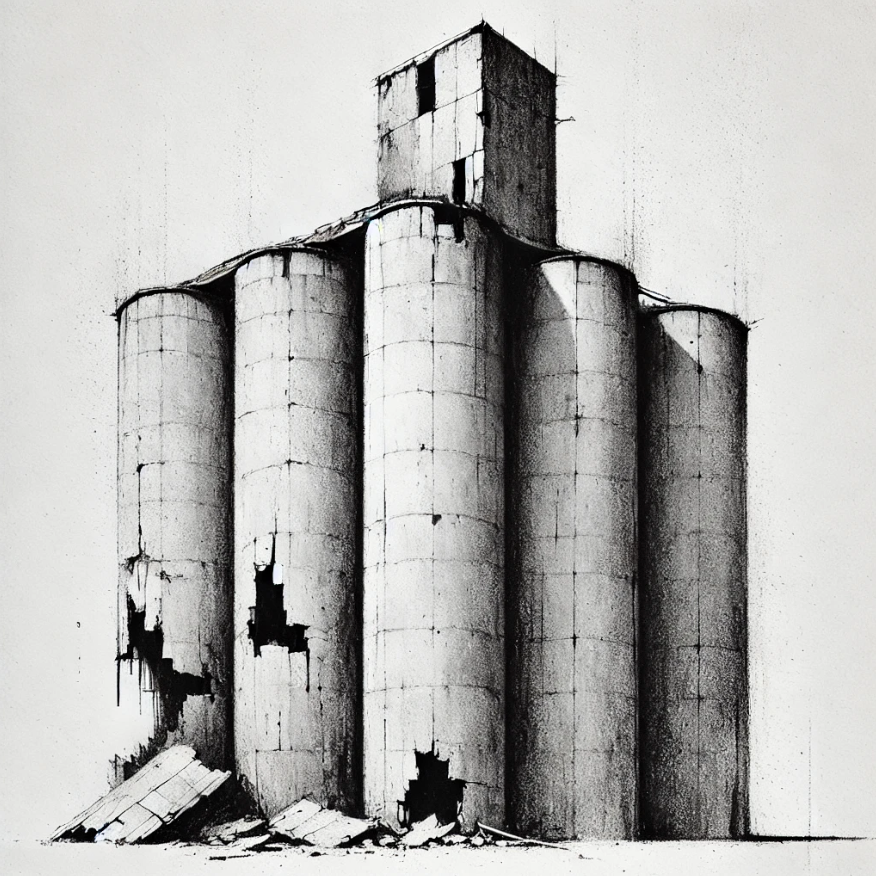
Breaking Down Silos:
Creating Cross-Functional AI Teams
In the rush to adopt artificial intelligence, organizations often overlook a fundamental truth: AI transformation is not merely a technical challenge—it's an organizational one. Across industries, from government agencies to Fortune 500 companies, leaders are discovering that their carefully constructed departmental structures, once designed for efficiency and specialization, have become barriers to effective AI implementation.
These organizational silos, built over decades of traditional operations, create invisible walls that separate data, talent, and insights. A data science team might develop sophisticated algorithms without access to crucial domain expertise. IT departments might maintain valuable data assets without understanding their potential for AI applications. Meanwhile, business units continue operating with limited awareness of how AI could transform their processes.
The cost of maintaining these silos extends far beyond missed opportunities. Organizations waste resources on duplicated efforts, struggle with inconsistent data practices, and fail to leverage their collective intelligence. More critically, siloed approaches to AI development often result in solutions that solve symptoms rather than core business problems.
Creating cross-functional AI teams isn't just about bringing different skills together—it's about fundamentally reimagining how organizations operate in the age of AI. These teams serve as bridges across traditional boundaries, fostering environments where technical expertise meets domain knowledge, where innovation meets practicality, and where organizational learning accelerates exponentially.
This article draws from real-world experiences in building and leading cross-functional AI teams across complex organizations. We'll explore practical strategies for breaking down silos, establishing effective cross-functional teams, and creating the collaborative environment necessary for successful AI initiatives. Whether you're just beginning your AI journey or looking to optimize existing teams, understanding these principles will be crucial for navigating the challenges ahead.
The Cost of Organizational Silos
In the race to implement AI capabilities, organizations often overlook how deeply organizational silos can undermine their efforts. While the impacts may not always appear on quarterly reports, the true costs of maintaining these silos create ripple effects that can derail even the most promising AI initiatives.
-
Perhaps the most immediate and costly impact of organizational silos manifests in the realm of data management. When departments operate independently, they typically:
Maintain separate data repositories with inconsistent standards
Implement varying data collection and storage practices
Use incompatible systems that resist integration
Create redundant and often conflicting data sets
Consider a government agency where different departments maintain separate citizen interaction databases. The inability to create a unified view of citizen engagement not only hampers service delivery but also prevents AI systems from identifying patterns that could improve public services and resource allocation.
-
Siloed organizations frequently find themselves unknowingly funding parallel AI initiatives across different departments. This leads to:
Multiple teams solving the same problems independently
Inconsistent approaches to similar challenges
Redundant technology investments
Competing standards and frameworks
The financial impact extends beyond the immediate waste of resources. When organizations eventually attempt to integrate these parallel efforts, they often face substantial costs in reconciling different approaches and technologies.
-
Silos create invisible walls that block the free flow of ideas and expertise, resulting in:
Limited cross-pollination of insights between departments
Reduced opportunity for collaborative innovation
Slower adoption of best practices
Missed opportunities for synergistic solutions
When data scientists can't easily collaborate with domain experts, or when IT teams are disconnected from business units, the resulting AI solutions often fail to address core organizational needs effectively.
-
In regulated environments like government agencies or financial institutions, siloed approaches to AI development create particular challenges:
Inconsistent governance standards across departments
Difficulty maintaining comprehensive audit trails
Increased risk of regulatory violations
Challenges in maintaining consistent security protocols
These issues not only expose organizations to regulatory risks but also create technical debt that becomes increasingly expensive to address over time.
-
Perhaps the most insidious cost of organizational silos is their impact on organizational culture:
Development of department-specific languages and priorities
Resistance to cross-functional collaboration
Lack of trust between units
Reduced willingness to share resources and information
This cultural calcification makes it increasingly difficult to implement the collaborative approaches necessary for successful AI initiatives.
Case Study: The Hidden Costs of Siloed AI Development
A recent example from a large organization illustrates these costs in action. Three separate departments independently developed AI models for predicting system failures. Each team:
Used different data sources and methodologies
Developed unique validation approaches
Created separate implementation frameworks
Reached different conclusions from similar data
The result wasn't just tripled development costs - it created confusion among operators about which predictions to trust, leading to delayed maintenance decisions and increased system failures. The organization ultimately spent more resources reconciling these different approaches than it would have cost to develop a unified solution from the start.
The Compounding Effect
The true cost of organizational silos compounds over time. Initial inefficiencies in data management and resource allocation evolve into deeper structural problems that become increasingly expensive to address. Organizations find themselves paying an ongoing premium in:
Extended development cycles
Reduced adoption rates
Increased maintenance costs
Lost opportunities for innovation
Higher operational risks
The message is clear: in the age of AI, the cost of maintaining organizational silos is simply too high to ignore. Organizations must actively work to break down these barriers, not just to improve efficiency, but to create the collaborative environment necessary for AI initiatives to succeed.